How robots can orient themselves in an energy-efficient way
For robots to be able to move autonomously in space, they must be able to estimate where they are and how they are moving. Until now, this was only possible with a great deal of computing power and energy. A research team including ZHAW researcher Yulia Sandamirskaya has now developed a new type of energy-efficient solution and demonstrated its applicability to a real robot task. The results have been published in the renowned journal Nature Machine Intelligence.
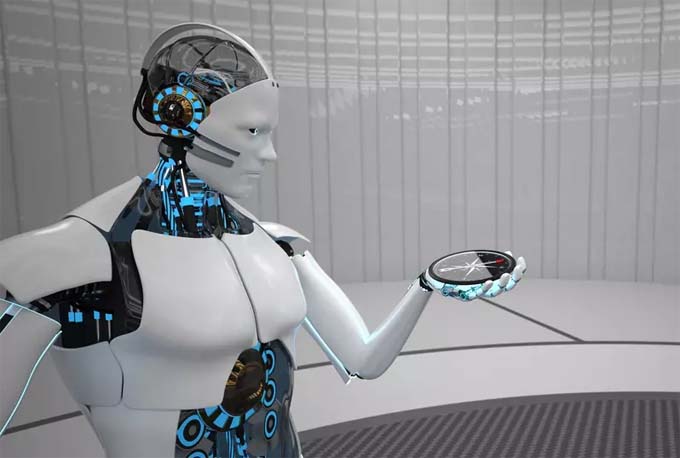
Even small animals such as bees, which have fewer than one million neurons, can easily find their way around complex environments. They use visual signals to estimate their own movement and track their position in relation to important locations. This ability is called visual odometry. In terms of compactness and energy efficiency, the solution that animals use is unmatched by the best current technical solutions for robots. However, for new applications such as small autonomous drones or lightweight augmented reality glasses to become possible, energy efficiency must be massively improved.
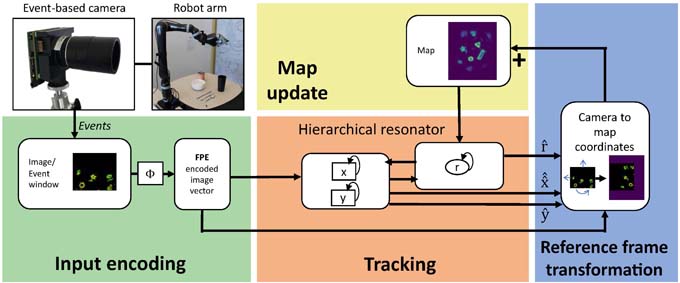
Built according to the example of natural neural networks
In the work published in Nature Machine Intelligence, an international team of authors propose a new neuromorphic solution, modeled on natural neural networks, which can also be efficiently implemented in neuromorphic hardware. The results presented represent an important step towards the use of neuromorphic computer hardware for fast and energy-efficient visual odometry and the associated task of simultaneous localization and mapping. The researchers have experimentally validated this approach in a simple robotic task and were able to show with an event-based dataset that the performance is state of the art.
More transparency in AI
The big difference to today's AI, such as that used by ChatGTP, is that the method described can put together or take apart various components of a visual scene to create a composition. The components include information such as "Which objects are in it?" or "Where are they located?" and many more. In conventional neural networks, these components are mixed together and cannot be disentangled. The new method can. This is crucial for developing modular and, above all, transparent AI systems.