Fantastic AI and how to use it sensibly
Artificial intelligence is omnipresent, even in the field of IT security. However, current AI applications are no longer sufficiently helpful for cybersecurity teams. Ontinue, an expert in Managed Extended Detection and Response (MXDR), identifies four tasks where the use of artificial intelligence brings significant benefits.
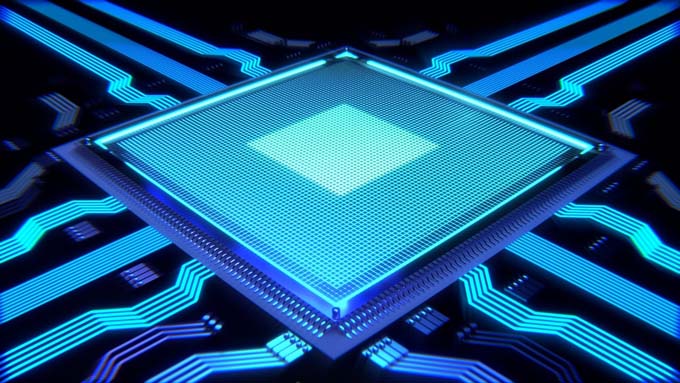
Without artificial intelligence and machine learning, IT would not be secure in any way today: cybersecurity teams and security analysts need digital helpers to identify viruses, malware, hackers and other threats. When it comes to threat detection, algorithms based on machine learning are showing their full strength and providing increasingly reliable warnings of cyberattacks and virus attacks. They are now so good that they can even recognize potential threats and issue a warning. However, these sophisticated AI capabilities are a double-edged sword: if AI is only used for threat detection, the countless warnings can quickly lead to alert fatigue. Analysts are then no longer able to thoroughly check all notifications and identify actual threats. In addition, on average only around ten percent of the threats found by modern algorithms are actually a cause for concern, meaning that processing all alerts would be a waste of time even if the team theoretically had the necessary capacity to do so. According to Ontinue, it is better for cybersecurity teams to use AI for the following tasks in addition to threat detection:
- Criticality assessment of IT assets
IT infrastructures in companies are becoming increasingly complex and are constantly changing. For many cybersecurity teams, it is therefore increasingly difficult to maintain an overview and keep a list of business-critical IT assets, i.e. the hardware and software systems used in the company. Machine learning applications can help to identify these security-relevant parts of the IT infrastructure, for example on the basis of which systems they are connected to, how and by whom they are used and for which processes they are required.
- Improvement of incident handling
The correct prioritization of an incident can determine whether a "true positive" alarm, i.e. a real threat to security, is detected and processed in time. Using machine learning to analyze and prioritize potential threats based on different factors can significantly improve security. For example, an AI would check an incident to see whether it could be dangerous for the IT environment used by the company. If this is not the case, it is a so-called benign positive - a security incident that cannot cause any damage and therefore does not require a response from the cybersecurity team.
- Automation of security measures
Without machine learning, the automation of responses to security incidents is not feasible. With the help of machine learning, patterns can be identified that are dealt with as standard in frequently occurring security incidents. Based on this information, cybersecurity teams can then define automated responses to these incidents.
- Vulnerability management
Artificial intelligence also comes into its own in vulnerability management: In this context, too, security experts can use machine learning to feed AIs with information from previous attacks. The algorithms then automatically recognize which vulnerabilities have been exploited and warn users about the gaps in the system that are most likely to be targeted by hackers. In this way, cybersecurity teams can prioritize the elimination of precisely these vulnerabilities.
"The democratization of artificial intelligence has not only advanced cybercriminals," explains Theus Hossmann, Director of Data Science at Ontinue. "Of course, hackers and now even laypeople can use generative AI tools to write malware. But defenders also benefit from increasingly powerful algorithms and machine learning capabilities - but they have to use them wisely: AI-based threat protection is just one piece of the puzzle in a holistic cybersecurity strategy."
Source: www.ontinue.com