Embedded AI relieves the burden on service
Complex production machines are costly and at the same time the basis for a company's turnover. According to estimates, the cost of a machine failure can be up to several hundred thousand CHF per hour[1]. Viacheslav Gromov, founder and CEO of AITAD comments on the situation in Switzerland and explains how embedded AI relieves the service and creates space for new innovative business models.
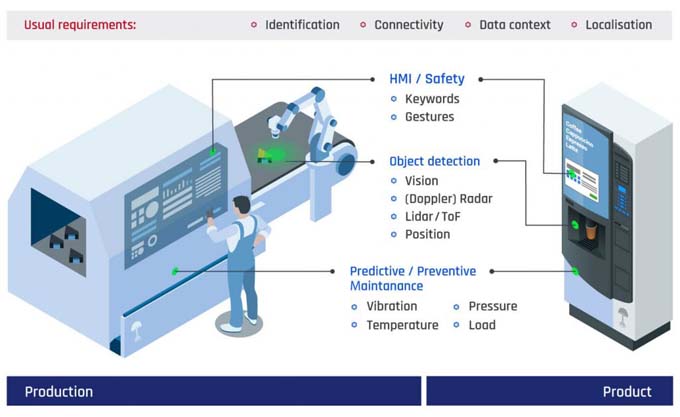
Machine builders rely on regular service to ensure that the machines are running, and that the quality and throughput are right. However, regular maintenance intervals are personnel-intensive - and it is not always easy to find suitably qualified personnel in the first place. And not every maintenance call is necessary: If the machine is running smoothly, the service technician's visit was basically a waste of time.
Reduce service intervals
In the past, regular service intervals were indispensable - and yet no guarantee that a machine would not suddenly come to a standstill. Even for highly qualified personnel, it was not possible to detect every failure that was already imminent.
This is where artificial intelligence comes into play. With the help of sensors, machines can be continuously monitored (predictive maintenance). This generates large amounts of data that can reveal a lot about the health of the machine or even individual components. If the data from the sensors is now evaluated by an AI, possible failures can be predicted well in advance. This means a win-win situation for both the manufacturer and the user: Unplanned failures no longer occur despite a reduction in service intervals.
However, most AI solutions currently on the market require a lot of computing capacity and rely on a costly, high-performance network infrastructure. The sensor data is transmitted via the network, analyzed on central servers or in the cloud, and then the result is sent back. This not only costs time, but also raises the issue of data security. Of course, it is an understandable manufacturer interest to access this data - but whether the customers who use the machines want potentially sensitive process data to be transmitted over the Internet remains to be seen.
AI solutions do not have to be complex and expensive
With modern, yet inexpensive sensors, it is now possible to acquire data in the gigasample range. With this amount of data - we are quickly talking about a volume of several terabytes, especially if the data from several machines is to be evaluated - transmission in the network is hardly possible, even with direct fiber optic cabling. Therefore, it is not only more clever, but also significantly more cost-effective to perform the data evaluation directly on site in the device.
However, such local monitoring has only been possible for a few years. The increasing computing capacity of even the smallest semiconductors makes it possible to accommodate the sensor and AI directly on a small circuit board adapted to the installation location. The sensor then no longer sends its data via the network, but directly to the AI on the same board. The AI "looks" at every single snippet of data in RAM, evaluates it, and then discards the raw data from the sensor. Only the evaluation results are passed on - in the simplest case by a lamp on the device that lights up red if an error occurs. Or the service department is notified directly that a component or the entire device will have a fault within a certain period of time. The service department can now order the necessary spare parts and plan a maintenance date with the customer that will not affect production.
Such local AI systems are referred to as "embedded AI". Due to the system-related resource limitations, such systems are comparatively inexpensive without sacrificing robustness. They do not incur any follow-up costs such as those incurred by a network infrastructure and are also real-time capable, which means they can also be used in safety-critical environments.
What are the possible applications for embedded AI
Even though the possible applications for embedded AI are almost unlimited, a (small) selection of potential use cases will be presented here:
- In engines, drive shafts can be monitored using ultrasonic sensors. If anomalies occur in the ultrasound image that indicate a possible failure, this is detected by the AI.
- Pumps and hoses can also be monitored with AI - it becomes visible at an early stage whether a hose is about to crack, for example. The AI can even detect whether, for example, the original detergent or a substitute is flowing through the hose.
- Axles and dampers can be monitored e.g. by means of pressure, vibration or even sound sensors
- Wear of conveyor belts can be detected at an early stage by means of spectrography
- Monitoring of main failure components and or wear parts in machines
- Monitoring of cooling systems and heating elements
Failure guarantee despite less service and win-win for manufacturer and customer
For service, predictive maintenance means that service intervals can be reduced and "unnecessary" visits avoided, and the planning of maintenance work can be made significantly more flexible. Machine manufacturers can guarantee a high level of reliability with fewer service personnel.
These new possibilities of predictive maintenance open up further potential for the machine manufacturer "quite incidentally". In addition to service reduction, it is not only possible to guarantee reliability; this scenario is also suitable for opening up a new business model: leasing machines instead of selling them. The interest in obsolescence decreases because the longest possible service life becomes a market advantage for the manufacturer. This also has advantages for the customer: machine procurement does not become a large one-time investment and is less of a burden on liquidity.
Especially in times of personnel shortages and sustainability efforts, machine builders can kill several birds with one stone with embedded AI and build another USP. In this way, they become reliable partners in the long term and at the same time set themselves apart from mass products, for example from Asia.
Author:
Viacheslav Gromov is founder and managing director of AITAD. The company develops electronics-related artificial intelligence (embedded AI) that performs locally defined tasks in devices and machines in real time. He is the author of numerous papers as well as various textbooks in the semiconductor field.